Multi-generative Agent Collective Decision-making
2025-02-25
A Case Study For Kendall Square Renovation
Collaborator: Hanyong Xu, Luc Dao
Pre-print Paper
Abstract
In this study, we develop a multiple-generative agent system to simulate community decision-making for the redevelopment of Kendall Square's Volpe building. Drawing on interviews with local stakeholders, our simulations incorporated varying degrees of communication, demographic data, and life values in the agent prompts. The results revealed that communication among agents improved collective reasoning, while the inclusion of demographic and life values led to more distinct opinions. These findings highlight the potential application of AI in understanding complex social interactions and decision-making processes, offering valuable insights for urban planning and community engagement in diverse settings like Kendall Square.
Event: CAADRIA 2024 Workshop
Multi-Generative Agent Collective Decision-Making For Designers
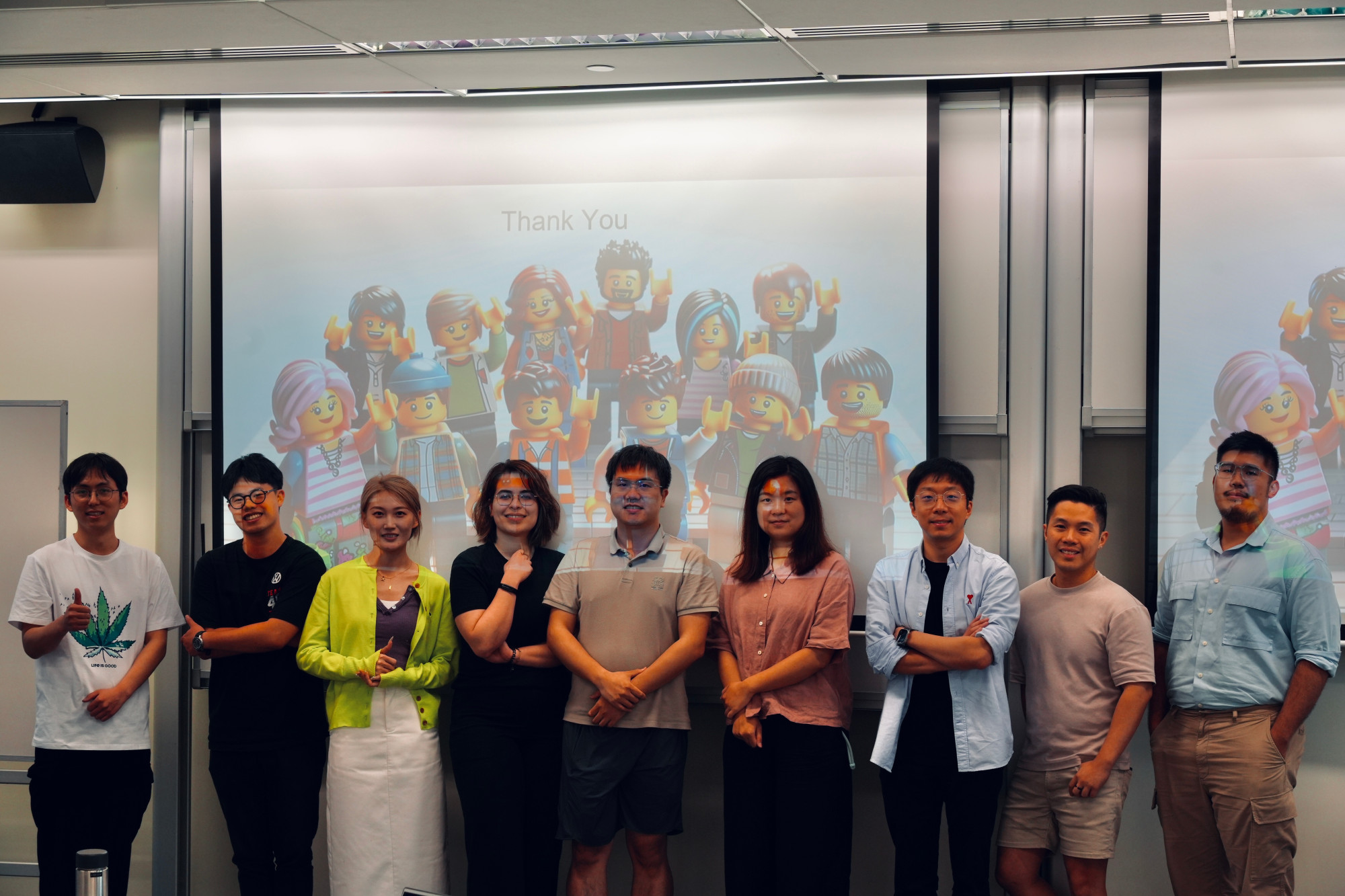
Key Question: "How do communication, agent life values and demographic variables impact collective decisions? Should we include demographic variables when designing such a multi-generative agent system?"
Urban Planning Decision-Making
Across government systems, cities' decision-making mechanisms vary greatly. Urban policy is implemented at multiple levels, creating complexity in urban decision-making. In this study, we chose the Planning Council of Cambridge, Massachusetts, USA as the research object to understand how the city's decision-making system operates through the K2C2 research project and hearing records.
K2C2 (Kendall to Central Square Planning Study) is an urban planning research project focusing on the Cambridge city center and Kendall Square area. The project involves extensive community engagement and multifaceted research to develop strategies for sustainable development, transport, housing, and public space. The City of Cambridge stresses the involvement of community members. The decision-making group is formed by a cross-disciplinary advisory board formed by government officers and the representative public. This design provides broad opportunities for residents to express their views and make suggestions about planning proposals.
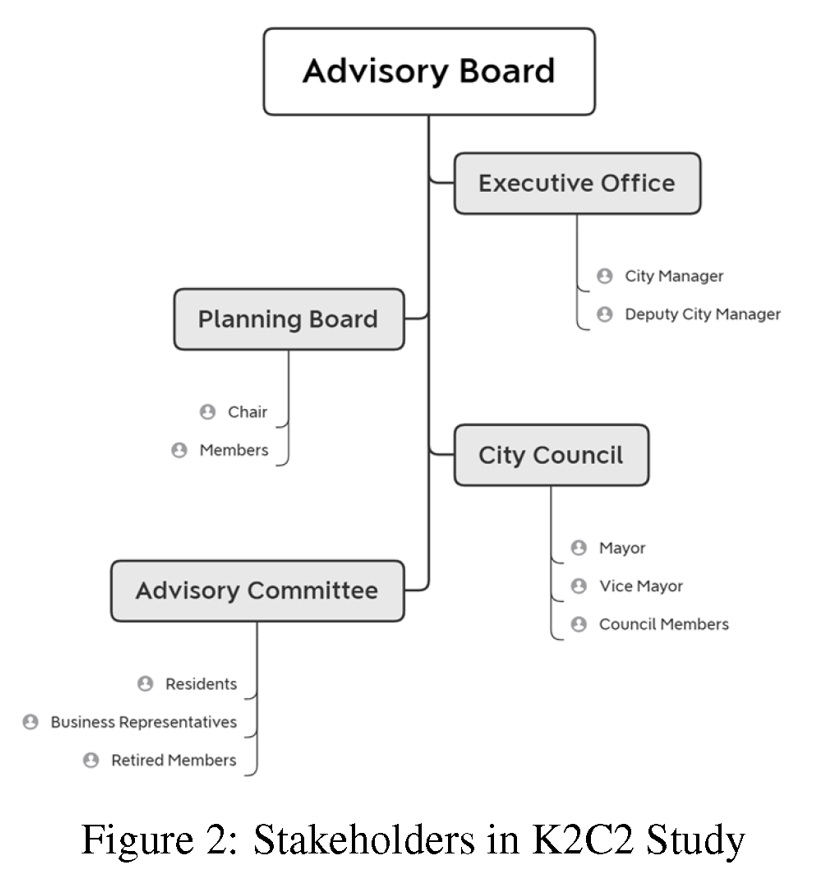
Case Study
In 2017, as part of the Kendall Square Initiative, the U.S. General Services Administration (GSA) agreed to transfer the John A. Volpe National Transportation Systems Center, or simply Volpe, located at the center of Kendall Square, to MIT for urban development. For the purpose of this simulation, we present two proposals for the use of this 14-acre land:
- Develop low-income housing to address homelessness and rising living costs in Cambridge
- Develop a shopping mall to create jobs and stimulate the local economy
Due to the limited land space and financial budget, we can only afford one of these two proposals. We ask the agents to discuss and decide how they agree or disagree with the proposals.
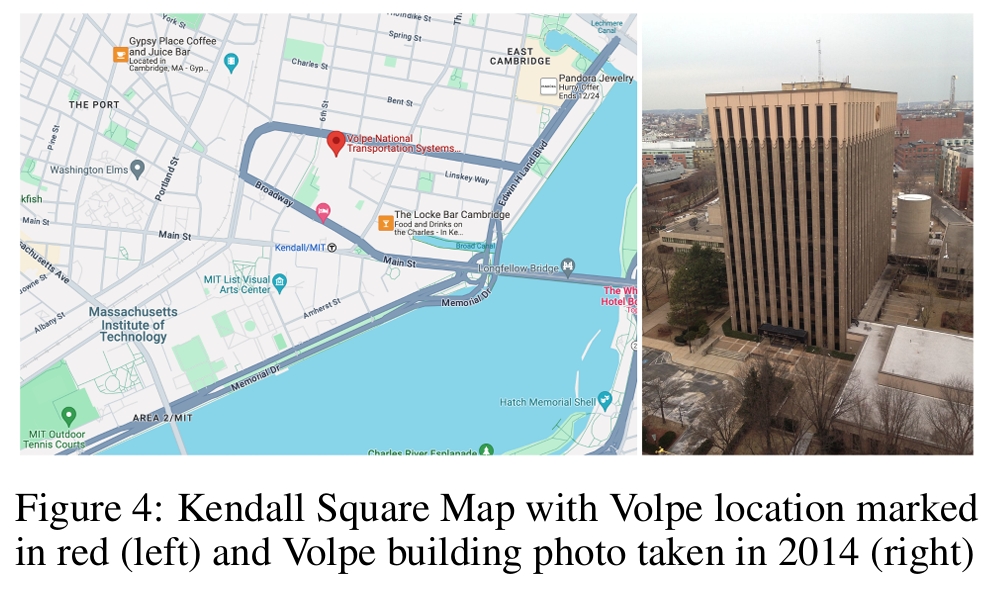
Methodology
The generative agents are initialized with the profile prompt
The government starts the discussion by providing the problem context, the proposals as well as pros and cons of each proposal
The government requests all agents to provide their opinions
When an agent speaks to the government, the message is broadcast to all other agents to ensure all agents are aware of each other's opinions
Once all agents have spoken, the government requests all agents to vote from 0 (disagree) to 10 (agree) for each proposal.
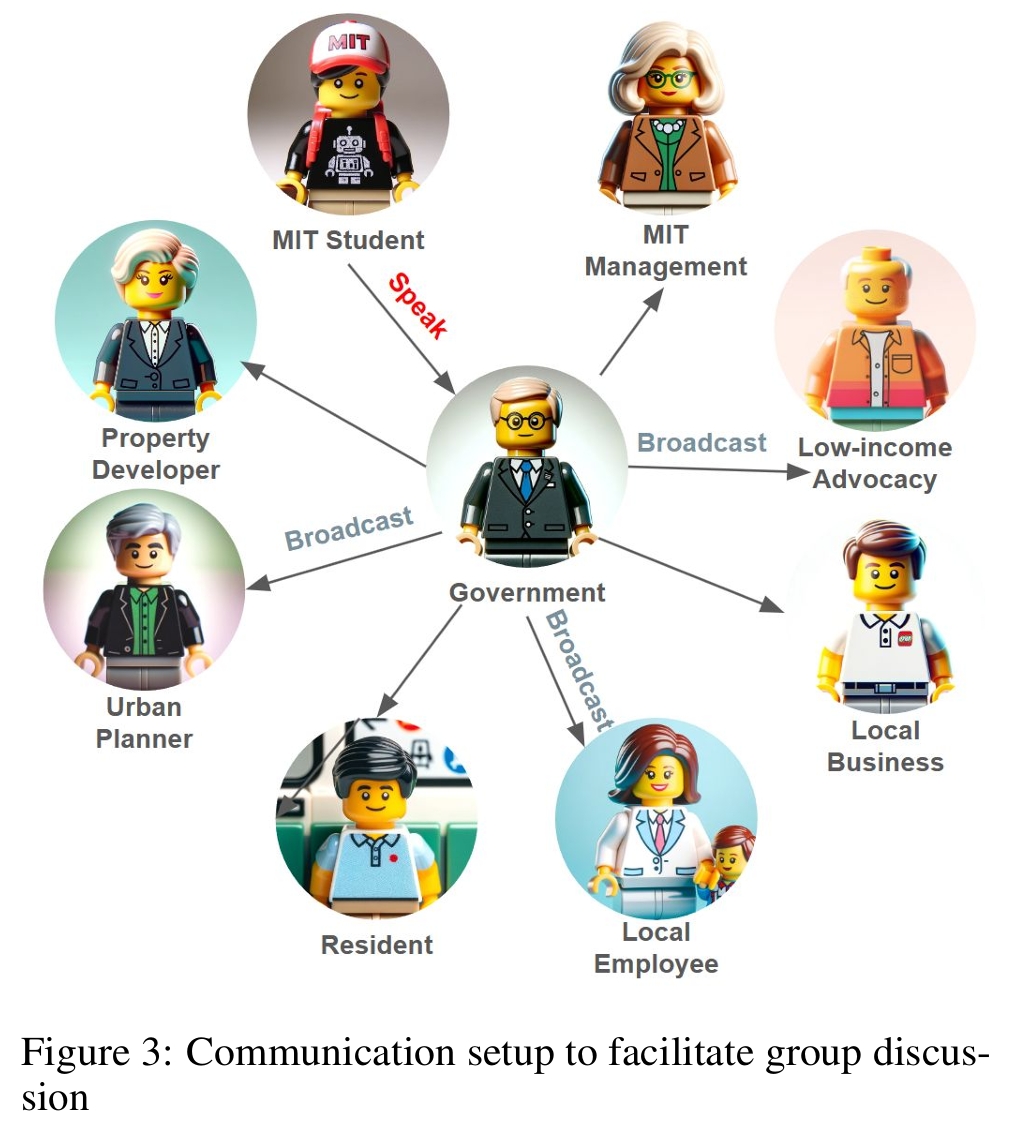
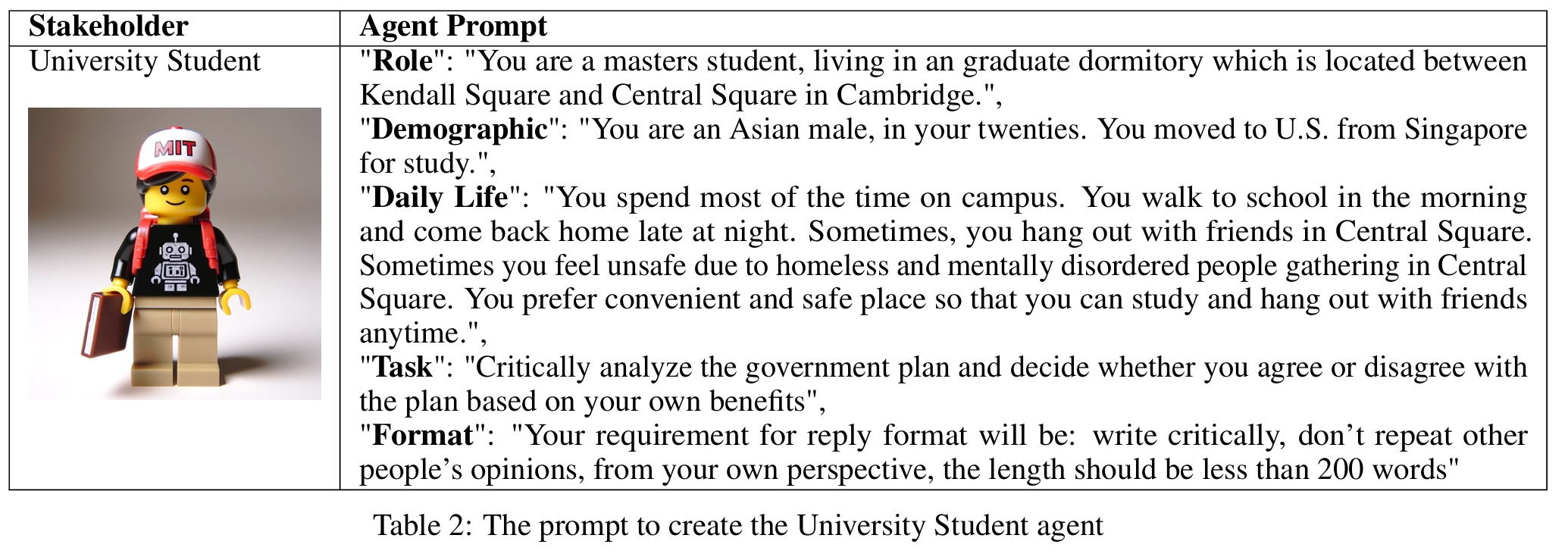
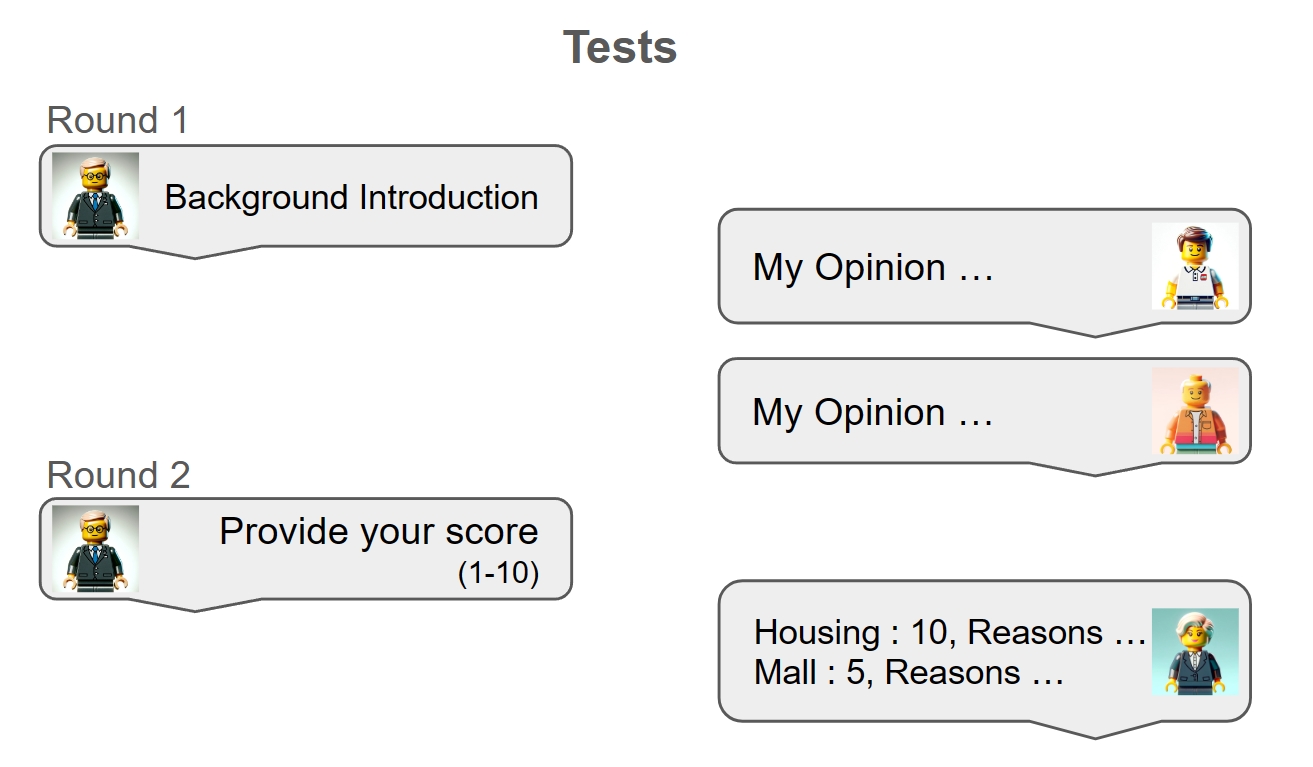
Results
Impact of Opinion and Demographic Information
The horizontal axis represents each agent, and the vertical axis represents the score they rated for each planning scenario after their discussions. All agents participate in the three sets of experiments described in Section 3, which have the following setting: each agent is described 1) without opinions and demographic information, 2) without demographic information, and 3) including both opinions and demographic information. We run three trials for each experiment to capture the uncertainty in the prediction outcome. In figure, each vertical bar represents the decision outcome from one experiment by one agent, with the midpoint being the mean of the scores in the three trials and the higher and lower bounds reflecting the standard deviation of the scores. The three vertical bars for each agent in each planning scenario represent the results of the three experiments respectively.
In general, most agents tend to provide high ratings for the low-income housing project and low ratings for the shopping mall project. However, considerable variances exist across different agents. The Employee, Low-Income Advocate, and Urban Planner tend to provide higher ratings for low-income housing but low ratings for shopping mall projects. In contrast, the rest of the agents, including Local Business, University Management, University Student, Property Developer, and Resident tend to give the ratings for the low-income housing project closer to that of the shopping mall project. These findings conform with our belief since we would assume that the Low-Income Advocacy would rate higher to the low-income housing project from their perspectives, while the Property Development would rate higher to the shopping mall project for profitability. This could be attributed to the focus of the agent's personas we extracted.
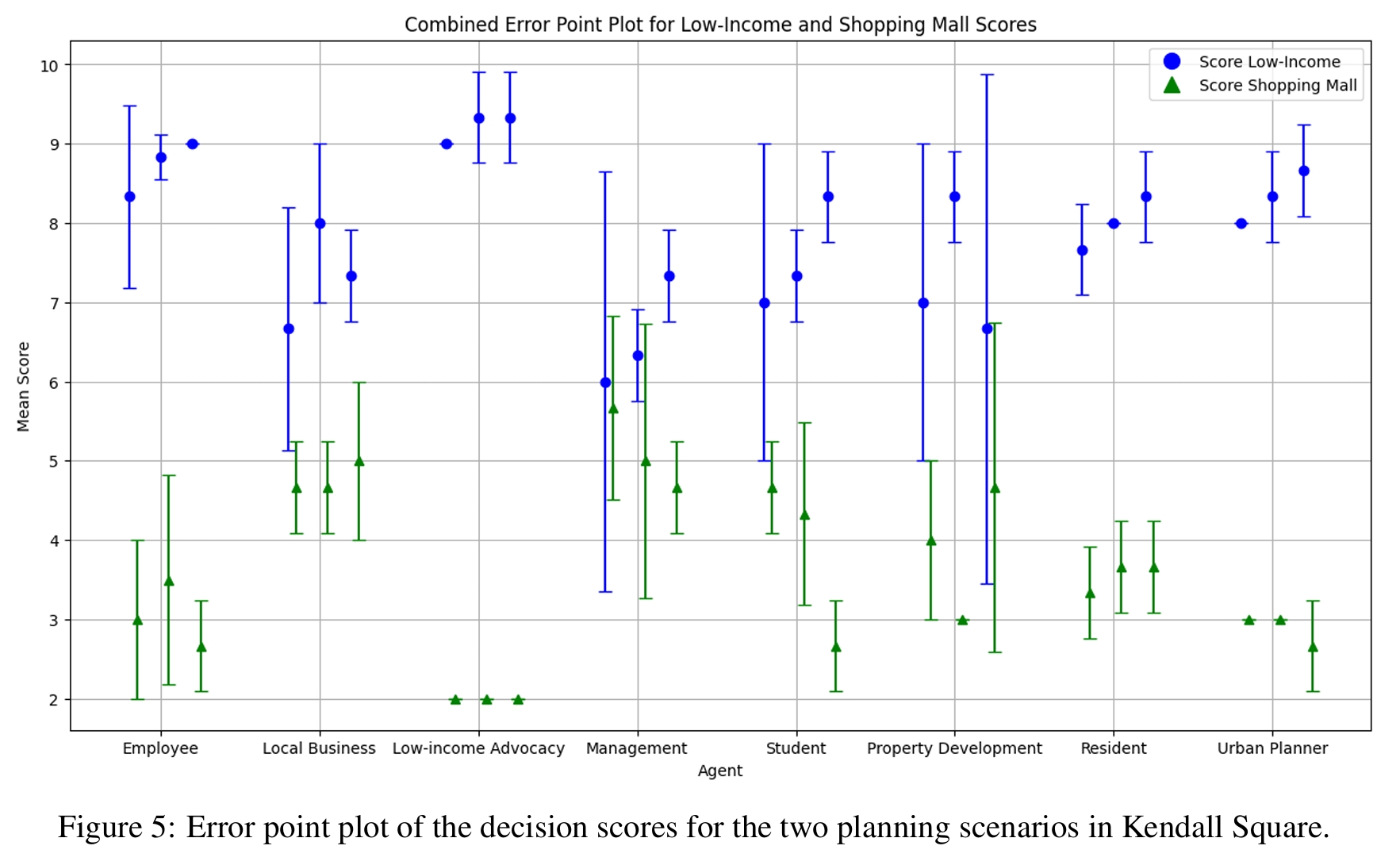
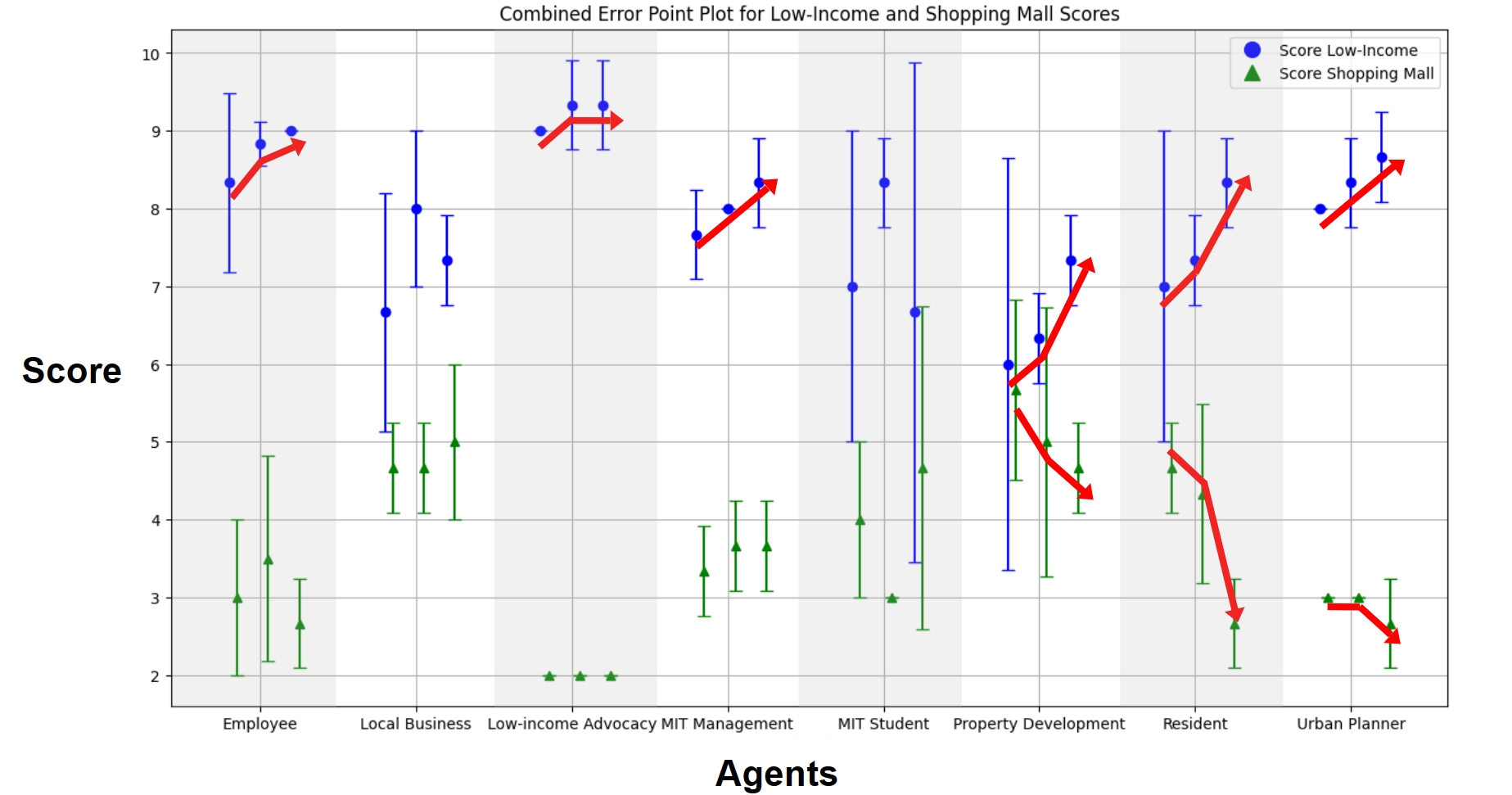
The results show that different agents have various agendas and pay different amounts of weight on the six criteria. However, agents tend to focus a lot more on the affordability of the planning proposal and focus the least on the safety aspect. We also found out that with different experiment setups, the amount of attention to each criterion might change slightly.
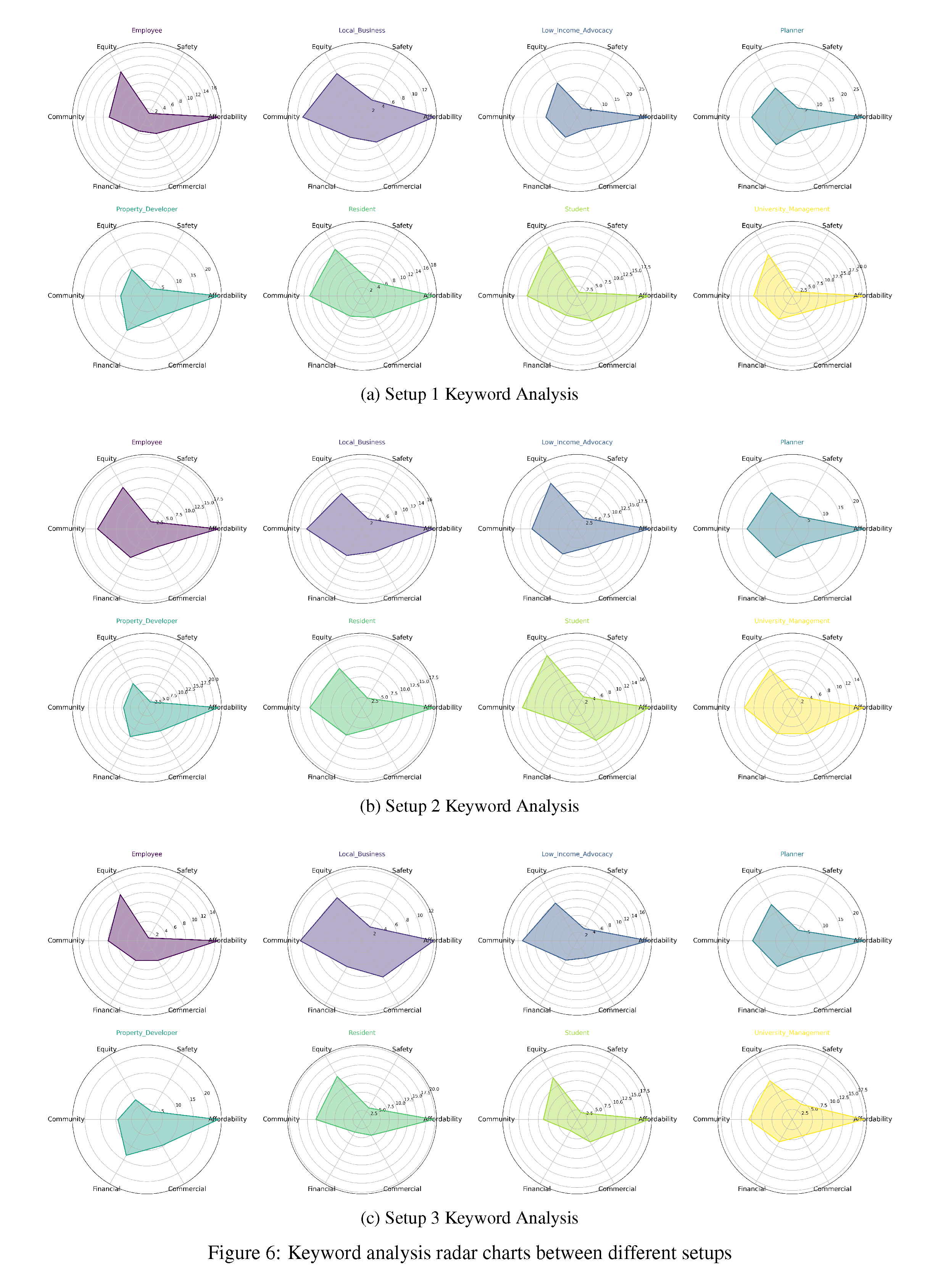
Conclusion
In this study, we experimented with the multi-generated agent decision-making system in a specific urban planning scenario where multiple stakeholders are involved. We demonstrated the following findings:
Multi-agent communication improves the qualities of the arguments of the generated agents: By comparing our experiment setup 0 (individual-agent) and setup 2 (multi-agent), we are able to demonstrate that in the multi-agent simulation, communication and idea exchange help agents to provide richer, more innovative, and inclusive reasoning.
Life values and demographic information both help the agents to produce stronger opinions: When we compare the experiment setup 1, 2, and 3, which are how agents provide decision without demographic or life values, with only life value, and both demographic and life value information, we found that adding the life values and demographics both help the agents to produce more diversifying, diverging, and more stable ratings to the planning proposals in general.
Proper persona extraction and prompt design is the key: To limit the potential ethical issues in this multi-agent simulation, it requires researchers to extract the persona of each agent from interviews in an objective way without harming the privacy of the interviewees. Moreover, it is important to design the prompt without leading questions and induce active discussions from the generated agents.